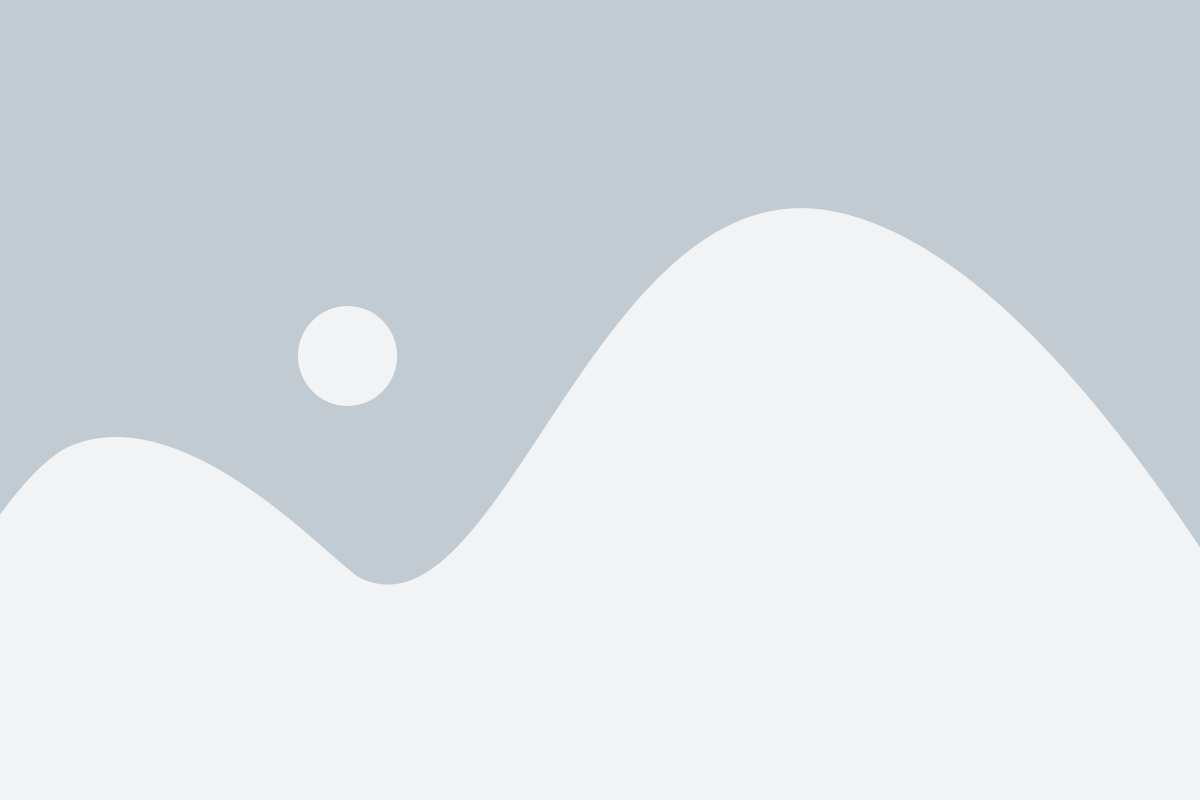
Artificial Intelligence (AI) and data science are transforming industries and improving decision-making. However, ethical concerns related to bias and fairness in AI models have emerged as critical challenges. As AI systems become more integrated into daily life, ensuring ethical and unbiased algorithms is essential to fostering trust and fairness.
- Understanding AI Bias
Bias in AI occurs when a model systematically favors or discriminates against certain groups due to skewed data, flawed algorithms, or human prejudice embedded in the training process. Bias can arise from various sources, including:
- Historical Data Bias: AI models trained on historical data may learn and perpetuate existing societal inequalities.
- Sampling Bias: If training datasets are not diverse, AI models may not generalize well to different populations.
- Algorithmic Bias: Some machine learning techniques can amplify small biases in data, leading to significant disparities.
- Confirmation Bias: AI models may reinforce pre-existing assumptions in data rather than uncovering new, objective insights.
- Real-World Impacts of Bias in AI
AI bias can lead to serious consequences in various domains:
Hiring and Recruitment
AI-driven hiring tools may inadvertently favor specific demographics if trained on biased hiring data. For example, a system trained on past hires may disadvantage women or minority candidates if historical hiring was not diverse.
Healthcare Disparities
Bias in medical AI models can lead to inaccurate diagnoses for underrepresented populations, potentially worsening healthcare inequalities.
Criminal Justice
Predictive policing and risk assessment tools may disproportionately target marginalized communities if trained on biased law enforcement data.
Financial Services
AI models used in lending and credit scoring may reject applicants from certain socioeconomic backgrounds due to biased historical data.
- Ensuring Fairness in AI Models
To mitigate bias, data scientists and AI researchers must adopt fairness-focused practices:
Diverse and Representative Datasets
Using inclusive datasets that reflect various demographics helps prevent AI models from reinforcing existing biases.
Bias Audits and Fairness Metrics
Regular audits of AI models using fairness metrics can detect and reduce bias. Techniques such as disparate impact analysis and demographic parity can help assess fairness.
Explainability and Transparency
AI models should be interpretable, allowing stakeholders to understand how decisions are made. Transparent AI helps identify and rectify biased outcomes.
Ethical AI Frameworks
Organizations should establish ethical AI guidelines to ensure responsible AI development and deployment. Regulatory bodies and industry standards can play a role in enforcing fairness.
Human Oversight
AI decisions should not be made in isolation. Human-in-the-loop systems ensure that AI recommendations are validated by human judgment, especially in high-stakes applications.
- The Role of Regulations and Policies
Governments and organizations worldwide are developing policies to address AI bias and fairness. Key initiatives include:
- EU AI Act: The European Union’s proposal for AI regulations to ensure transparency, accountability, and fairness.
- Algorithmic Accountability Act (USA): A proposed legislation requiring companies to assess and mitigate bias in automated decision systems.
- Industry Guidelines: Companies like Google, Microsoft, and IBM are implementing AI ethics principles to guide responsible AI practices.
- The Future of Ethical AI
As AI evolves, addressing bias and fairness will remain a priority. Future advancements in AI ethics may include:
- Better Bias Detection Tools: AI-driven methods to automatically identify and mitigate bias.
- More Inclusive AI Training Data: Initiatives to create diverse datasets that represent all demographics fairly.
- Stronger Regulatory Frameworks: Global cooperation to standardize AI ethics policies.
Conclusion
Ethical AI development is crucial for ensuring fair and unbiased decision-making in data science. Addressing bias requires a multi-faceted approach involving diverse data, transparency, human oversight, and regulatory frameworks. By prioritizing fairness, AI can be a force for positive societal change rather than reinforcing existing inequalities.